Federated Quantum-Based Privacy-Preserving Threat Detection Model for Consumer Internet of Things
Abstract:
The Internet of Things (IoT) has significantly impacted the evolution of consumer-oriented smart environments, primarily due to its capacity for transformative device-to-device communication. While this capability enhances user convenience and experience in the Consumer IoT sector, it also generates vast amounts of data. While beneficial for consumer insight and electronic optimization, this data is vulnerable to security breaches.
We focus on Machine Learning-based threat detection systems to address these challenges within the Consumer IoT. While effective in recognizing threats, these systems often overlook crucial privacy considerations, a critical aspect in the realm of consumer devices. To counter this, Federated Learning (FL) emerges as a promising solution for maintaining data privacy in Consumer IoT. However, FL faces its own challenges, especially when dealing with malicious clients. This paper addresses two primary challenges in Consumer IoT threat detection. First, we tackle an unaddressed issue in FL: the rigorous validation of its clients. The advent of quantum computing could render traditional validation techniques obsolete. We introduce a quantum-centric registration and authentication strategy to overcome this, ensuring stringent client validation in an FL framework. The second challenge involves protecting clients’ model weights within FL. We propose the integration of Additive Homomorphic Encryption into our model, offering a robust solution that secures the privacy of FL participants without compromising on computational efficiency. Our empirical results underscore the efficacy of our approach, achieving an average accuracy of 94.93% on the N-baIoT dataset and 91.93% on the Edge-IIoTset dataset, showcasing consistent and robust performance across diverse client configurations. This approach can potentially significantly improve the security and privacy landscape of Consumer IoT.
Abstract:
The Internet of Things (IoT) has significantly impacted the evolution of consumer-oriented smart environments, primarily due to its capacity for transformative device-to-device communication. While this capability enhances user convenience and experience in the Consumer IoT sector, it also generates vast amounts of data. While beneficial for consumer insight and electronic optimization, this data is vulnerable to security breaches.
We focus on Machine Learning-based threat detection systems to address these challenges within the Consumer IoT. While effective in recognizing threats, these systems often overlook crucial privacy considerations, a critical aspect in the realm of consumer devices. To counter this, Federated Learning (FL) emerges as a promising solution for maintaining data privacy in Consumer IoT. However, FL faces its own challenges, especially when dealing with malicious clients. This paper addresses two primary challenges in Consumer IoT threat detection. First, we tackle an unaddressed issue in FL: the rigorous validation of its clients. The advent of quantum computing could render traditional validation techniques obsolete. We introduce a quantum-centric registration and authentication strategy to overcome this, ensuring stringent client validation in an FL framework. The second challenge involves protecting clients’ model weights within FL. We propose the integration of Additive Homomorphic Encryption into our model, offering a robust solution that secures the privacy of FL participants without compromising on computational efficiency. Our empirical results underscore the efficacy of our approach, achieving an average accuracy of 94.93% on the N-baIoT dataset and 91.93% on the Edge-IIoTset dataset, showcasing consistent and robust performance across diverse client configurations. This approach can potentially significantly improve the security and privacy landscape of Consumer IoT.

%20(1).png)
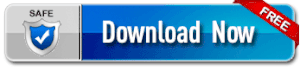
.png)
Không có nhận xét nào: